Resolution Techniques for NNs
There are two main challanges when it comes to running NNs which aren't in the same aspect ratio (AR) as the input frame:- Input frame AR missmatch - when your NN model expects a different aspect ratio compared to the sensors aspect ratio
- Visualization of the NN output - when you want to visualize the NN output on higher resolution
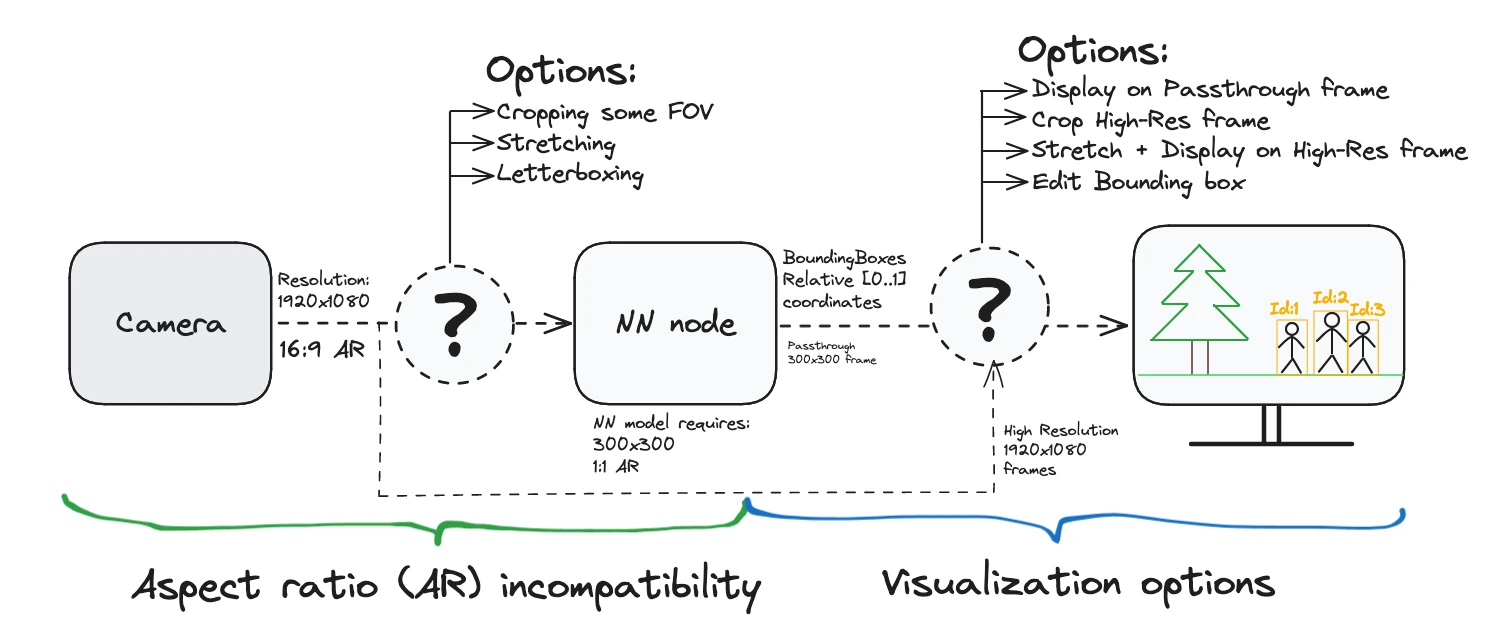
Input frame AR missmatch
A challenge occurs when your NN model expects a different aspect ratio (eg. 1:1) compared to the sensors aspect ratio (eg. 4:3), and we want to run NN inference on the full FOV of the sensor. Let's say we have a MobileNet-SSD that expects 300x300 input frames (1:1 aspect ratio), and we want to run inference on full FOV of the sensor - we have a few options:- Crop the ISP frame to 1:1 aspect ratio and lose some FOV
- Stretch the ISP frame to 1:1 aspect ratio of the NN
- Apply letterboxing to the ISP frame to get 1:1 aspect ratio frame
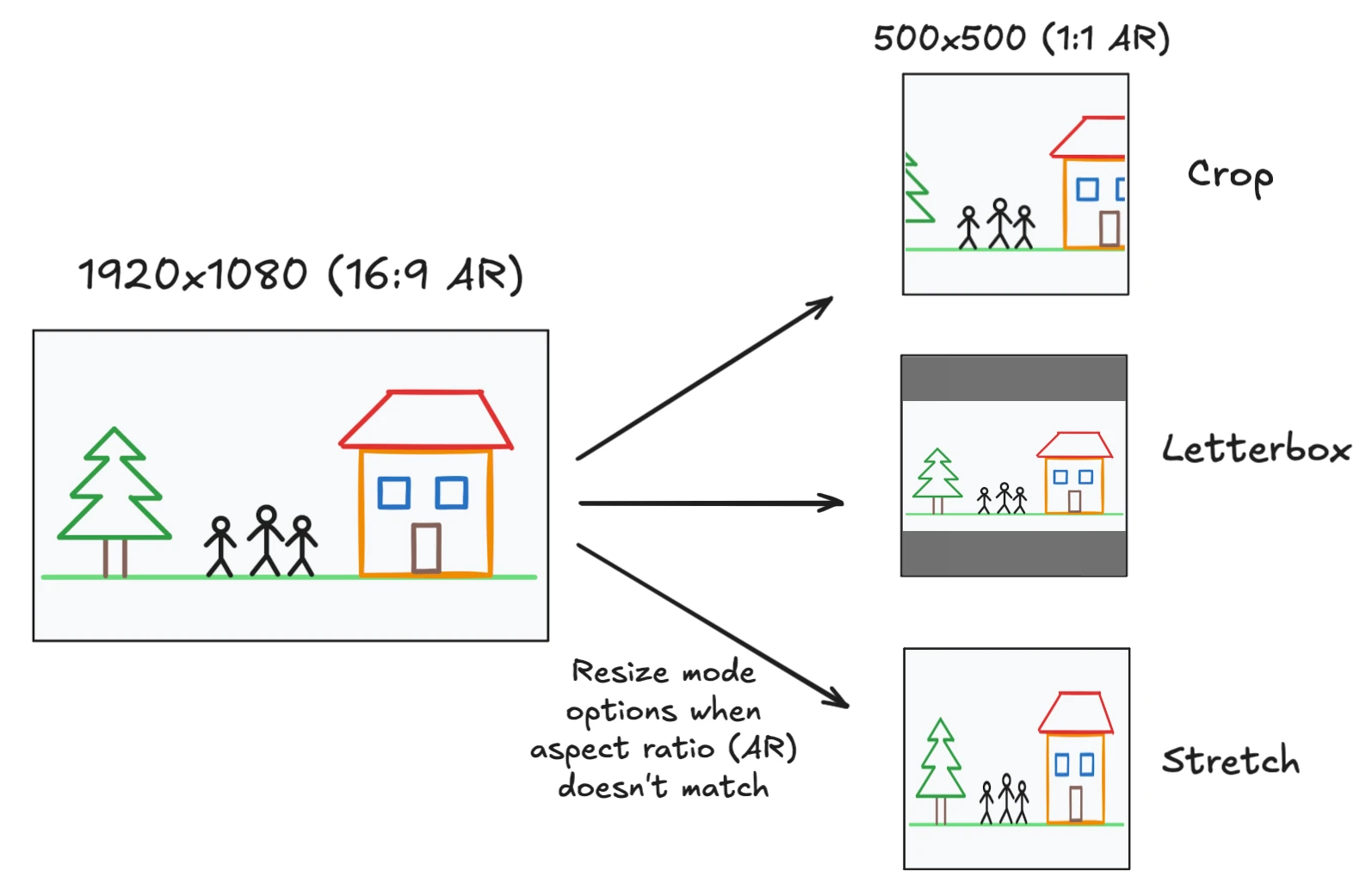
Crop
Pros: No NN accuracy decrease. Cons: Frame is cropped, so it's not full FOV.Cropping the full FOV (isp) frames to match the NN aspect ratio can be used to get the best NN accuracy, but this decreases FOV. Usage example here.Letterbox
Pros: Preserves full FOV. Cons: Smaller "frame" means less features might decrease NN accuracy.Letterboxing approach will apply "black bars" above and below the image to the full FOV (isp) frames, so the aspect ratio will be preserved. You can achieve this by using ImageManip withmanip.setResizeThumbnail(x,y)
. The downside of using this method is that your actual image will be smaller, so some features might not be preserved, which can mean the NN accuracy could decrease. Usage example here.Stretch
Pros: Preserves full FOV. Cons: Due to stretched frames, NNs accuracy might decrease.Stretching is done by changing aspect ratio. It can be configured withcamRgb.setPreviewKeepAspectRatio(False)
. This means the aspect ratio will not be preserved and the image will be "stretched". This might be problematic for some off-the-shelf NN models, so some fine-tuning might be required. Usage example here.Displaying detections in High-Res
To run an object detection model at realtime (~30FPS) on RVC2 you usually use lower input frames for inferencing (eg.300x300
or 416x416
). Instead of displaying bounding boxes on such small frames, you could also stream higher resolution frames (eg. video
output from ColorCamera
) and display bounding boxes on these high-res frames. There are several approaches to achieving that, and in this section we will take a look at them.Passthrough
Just using the small inferencing frame. Here we usedpassthrough
frame of MobileNetDetectionNetwork's output so bounding boxes are in sync with the frame. Other option would be to stream preview
frames from ColorCamera and sync on the host (or don't sync at all). 300x300
frame with detections below. Demo code here.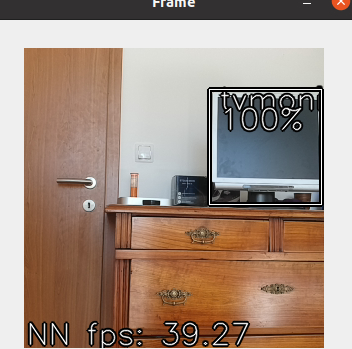
Crop high resolution frame
A simple solution to low resolution frame is to stream high resolution frames (eg.video
output from ColorCamera) to the host, and draw bounding boxes to it. For bounding boxes to match the frame, preview
and video
sizes should have the same aspect ratio, so 1:1
. In the example, we downscale 4k resolution to 720P, so maximum resolution is 720x720
, which is exactly the resolution we used (camRgb.setVideoSize(720,720)
). We could also use 1080P resolution and stream 1080x1080
frames back to the host. Demo code here.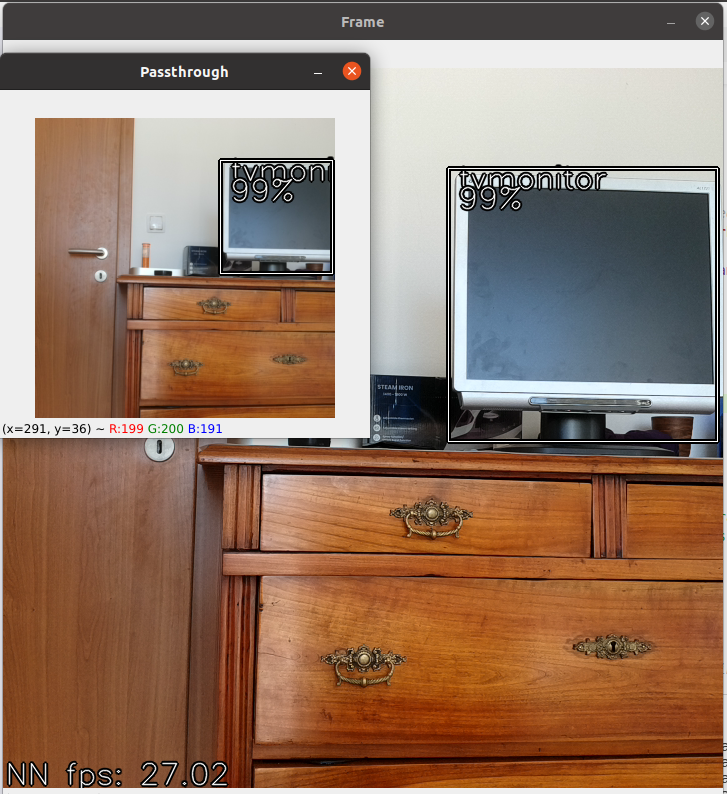
Stretch the frame
A problem that we often encounter with models is that their aspect ratio is1:1
, not eg. 16:9
as our camera resolution. This means that some of the FOV will be lost. Above (Input frame AR missmatch) we showcased that changing aspect ratio will preserve the whole FOV of the camera, but it will "squeeze"/"stretch" the frame, as you can see below. Demo code here.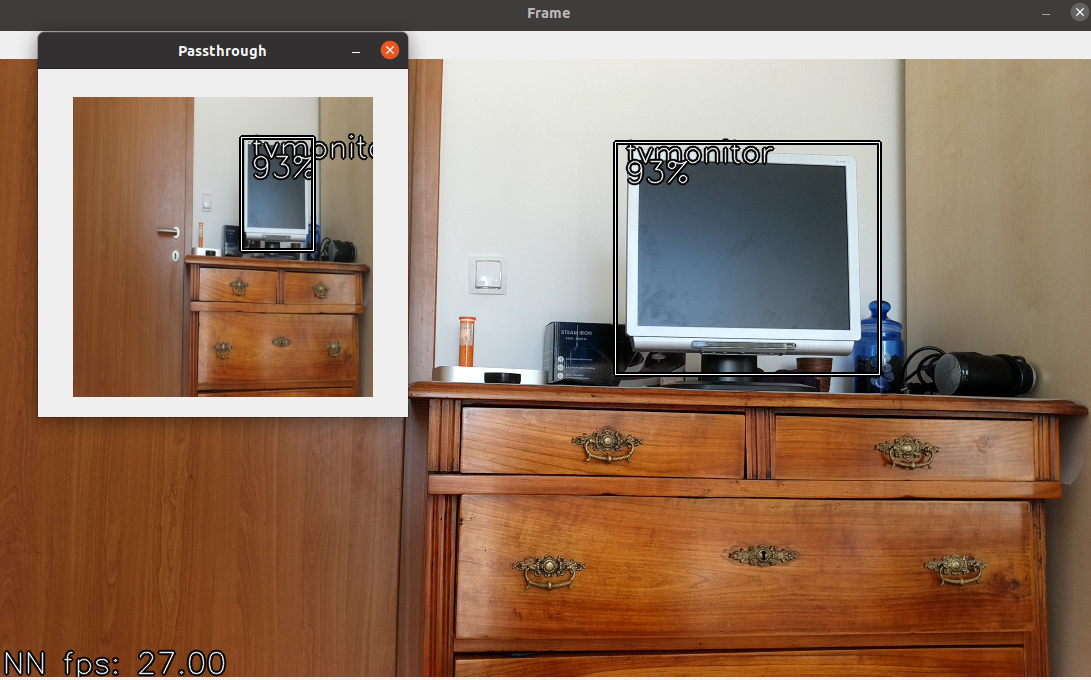
Edit bounding boxes
To avoid stretching the frame (as it can have an affect on NN accuracy), we could also stream full FOVvideo
from the device and do inferencing on 300x300
frames. This would, however, mean that we have to re-calculate bounding boxes to match with different aspect ratio of the image. This approach does not preserve the whole aspect ratio, it only displays bounding boxes on whole FOV video
frames. Demo code here.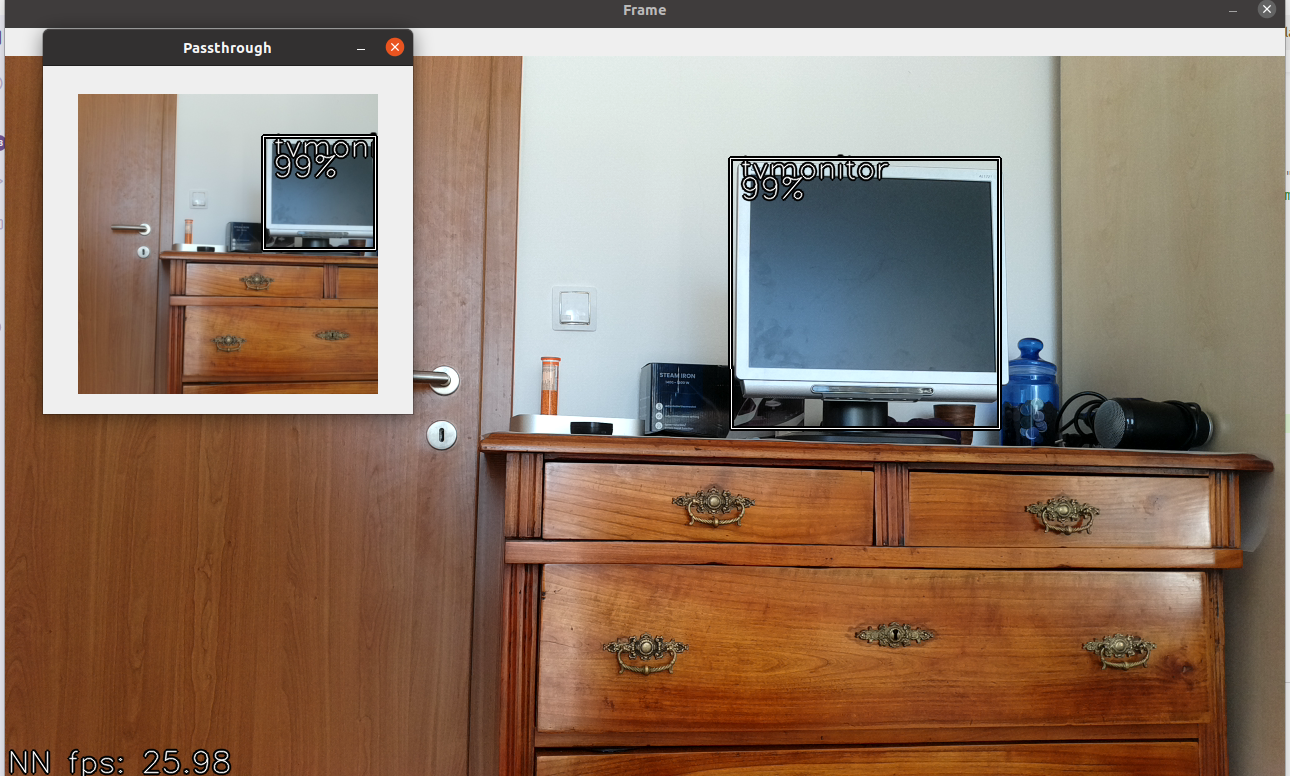